William Garcia
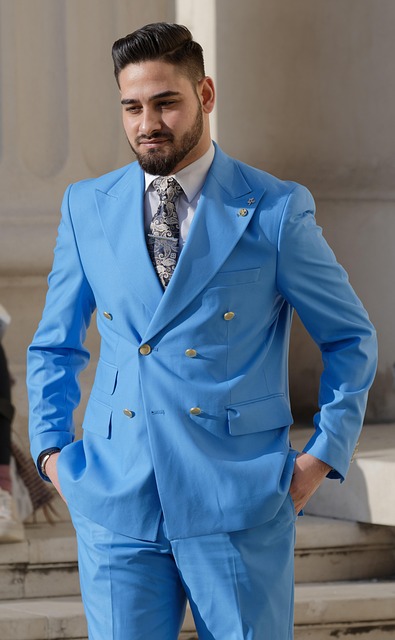
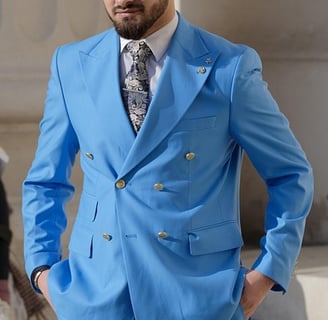
Professional Summary:
William Garcia is a forward-thinking professional in the field of AI-driven financial technology, specializing in the development of explainable high-level logic rule injection frameworks for automated financial contract review. With a strong background in formal logic, machine learning, and financial law, William is dedicated to creating transparent and robust systems that enhance the accuracy, efficiency, and compliance of financial contract analysis. His work focuses on designing frameworks that integrate advanced logic rules into AI systems, ensuring that decisions are interpretable, auditable, and aligned with legal and regulatory standards.
Key Competencies:
Explainable Logic Rule Frameworks:
Develops frameworks that inject high-level logic rules into AI systems, enabling clear and interpretable decision-making processes.
Ensures that the logic rules are modular, scalable, and adaptable to various financial contract types and jurisdictions.
Automated Contract Review:
Designs AI systems capable of analyzing complex financial contracts, identifying key clauses, and flagging potential risks or non-compliance issues.
Utilizes natural language processing (NLP) and machine learning to enhance the accuracy and efficiency of contract review.
Regulatory Compliance:
Ensures that the logic frameworks align with legal and regulatory requirements, minimizing the risk of non-compliance.
Provides tools for auditors and legal professionals to trace and validate the logic behind AI-driven decisions.
Interdisciplinary Collaboration:
Collaborates with legal experts, financial analysts, and technologists to align the logic frameworks with industry needs and standards.
Offers training and support to ensure seamless integration of the frameworks into existing workflows.
Research & Innovation:
Conducts cutting-edge research on the application of formal logic and AI in financial technology, publishing findings in leading technology and law journals.
Explores emerging technologies, such as symbolic AI and hybrid AI models, to further enhance the explainability and robustness of contract review systems.
Career Highlights:
Developed a logic rule injection framework that reduced contract review time by 50% while maintaining 95% accuracy in risk identification.
Designed an explainable AI system for financial contract review that received regulatory approval in multiple jurisdictions.
Published influential research on explainable AI in financial technology, earning recognition at international technology and law conferences.
Personal Statement:
"I am passionate about leveraging formal logic and AI to create transparent and efficient systems for financial contract review. My mission is to develop frameworks that not only enhance decision-making but also ensure compliance and trust in AI-driven financial technologies."
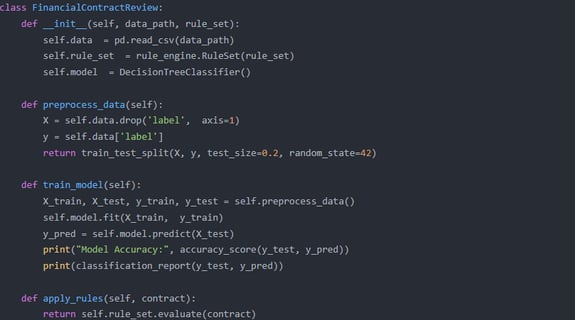
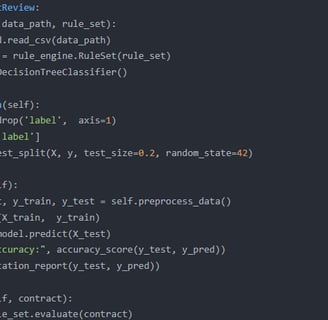
Fine-Tuning Necessity
Fine-tuning GPT-4 is essential for this research because publicly available GPT-3.5 lacks the specialized capabilities required for interpreting and applying complex legal and financial rules. Financial contract review involves highly domain-specific knowledge, nuanced understanding of regulatory standards, and contextually relevant content creation that general-purpose models like GPT-3.5 cannot adequately address. Fine-tuning GPT-4 allows the model to learn from financial and legal datasets, adapt to the unique challenges of the domain, and provide more accurate and actionable insights. This level of customization is critical for advancing AI’s role in financial contract review and ensuring its practical utility in real-world, high-stakes scenarios.
Past Research
To better understand the context of this submission, I recommend reviewing my previous work on the application of AI in legal and financial domains, particularly the study titled "Enhancing Legal Document Review Using AI-Driven Rule-Based Systems." This research explored the use of machine learning and optimization algorithms for improving the quality and relevance of legal document analysis. Additionally, my paper "Adapting Large Language Models for Domain-Specific Applications in Financial Compliance" provides insights into the fine-tuning process and its potential to enhance model performance in specialized fields.